2 min read
Managing Weather Damage w/Machine Learning to Benefit the Environment
Jacqueline L. Mulhern
:
5/16/22 10:05 AM
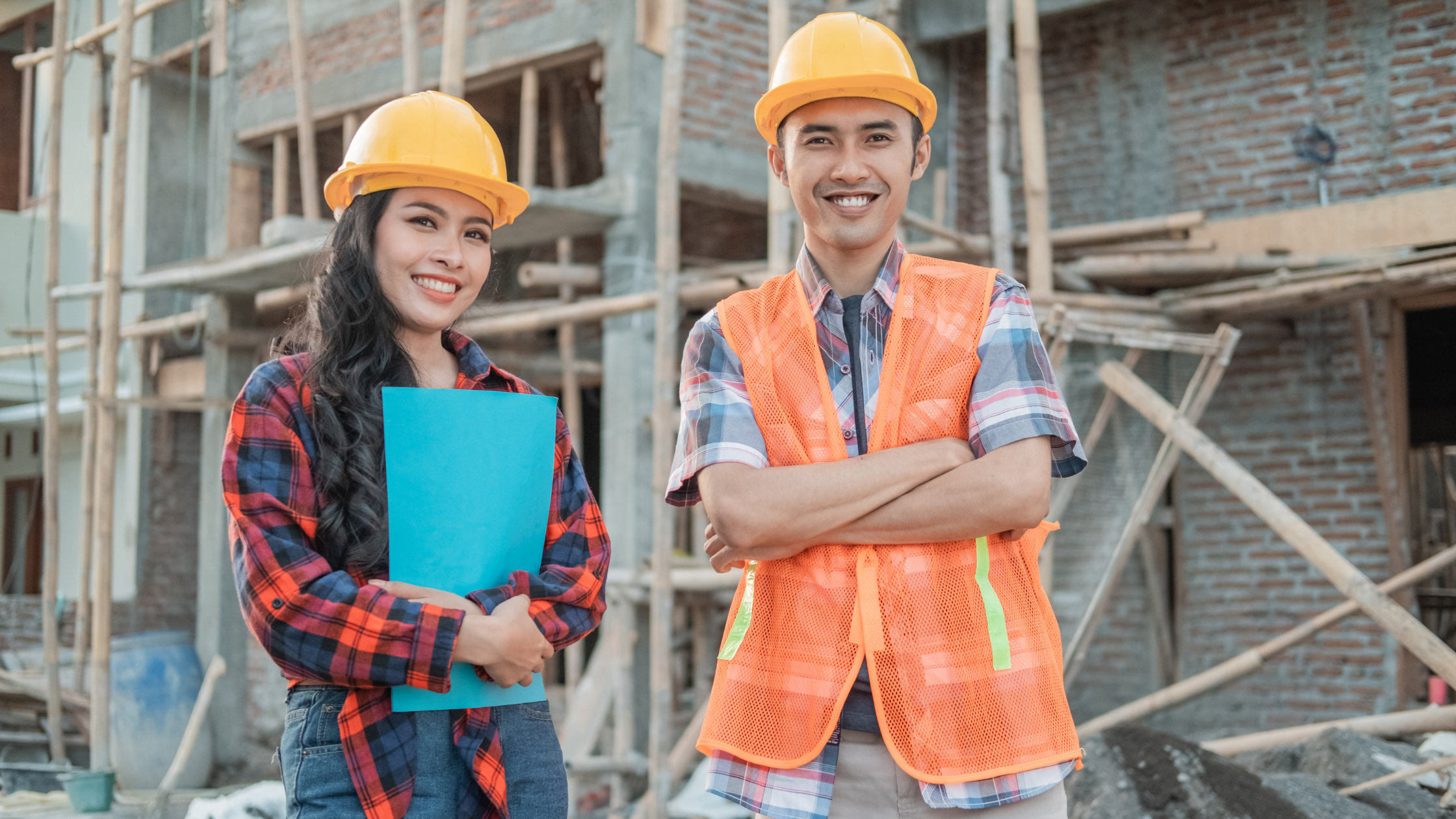
Seasonal weather conditions put tremendous strain on critical infrastructure in several industries. Data collection is optimized with digital tools that make assets in oil, gas, water, wastewater, and energy run more efficiently, reducing environmental pollution in the long term.
Real-time alerts sent to management quickly identify and address equipment repairs. Regulatory compliance becomes easier. The marriage of machine learning with renewable thermal energy reduces carbon emissions in the way that removing thousands of cars from the road benefits air quality. How does this work?
Renewable thermal energy reduces carbon emissions
One of the largest alternative energy companies in the United States provides steam, hot water, and chilled water for a clean source of energy known as district energy. (To learn more about district energy, read last week's article: District Energy Controls Your Office)
A vast network of underground pipes provides heating, cooling, humidification, sterilization, and other services to customers. It eliminates the need for boiler and chiller plants in individual buildings, improving efficiency, lowering carbon, and increasing reliability. This technology is more environmentally friendly than traditional heating & cooling systems.
The utility claims that district energy is not only more efficient, more reliable, and more resilient than a conventional onsite generation, but their clean steam has a lower carbon impact than other energy sources.
Automated data transmission
The power provider needed an accurate, secure way to transmit data. For several years, they have used the Ayyeka scheduler on top of a flow meter to track usage for billing purposes. They currently have over 750 flow meters installed in several states.
In the past, they sent operations staff to individually record the values from the flow meters, a process that was inefficient and time-consuming. The utility worked with Ayyeka to develop an automated data transmission process for their flow meters. The plug-and-play system was easy to install in the field and allowed for real-time data transmission to their databases.
Predictive maintenance means less downtime
AI embedded in edge devices provided valuable information to use for operations & billing purposes. On the billing side, it sped up the process and revealed missed opportunities in irregular systems. For operations, it allowed them to automatically send notifications when problems in their transmission network arise. They could then alert their customers of an interruption of service, some of whom (like hospitals and schools) needed to know these issues as quickly as possible. They could also be more proactive in the maintenance of their system as they could track conditions throughout the pipe network and monitor the conditions in their vaults. Predictive maintenance reduces down time of heating and cooling systems, allowing them to work more efficiently, a chief benefit of machine learning for the environment.
Conclusions
With a full billing and operations system set up, machine learning has also begun to build flow models of the utility's network, allowing them to have predictive analytics of their system, so they can fix it before it ever arises. As Ayyeka’s system comes fully inclusive of Sim costs and support, users have a seamless integration throughout the process, saving them time to focus on their operations, not on data retrieval.