2 min read
Data’s Like Garbage: How to Store It Right for Field Data Management
Jacqueline L. Mulhern
:
12/20/22 10:00 AM
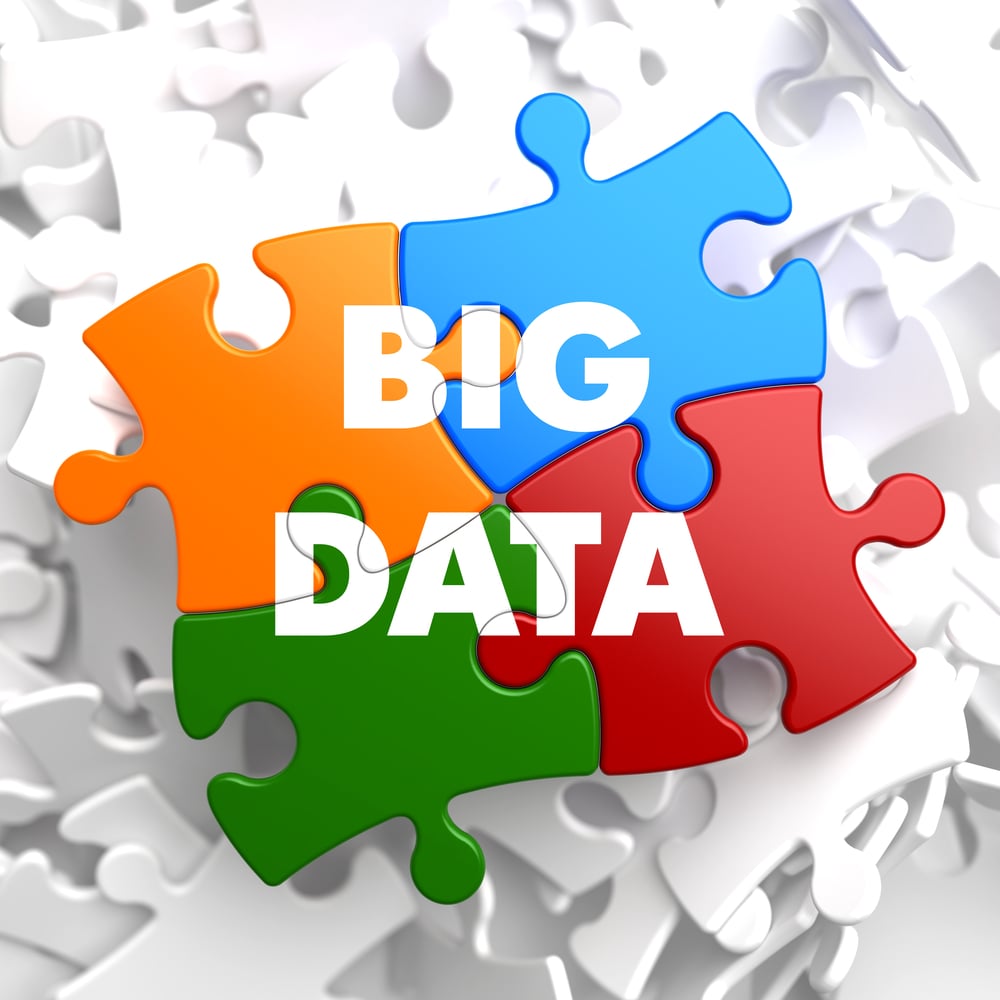
Digitalization of data is a process involving several steps. Executives who want to achieve the maximum benefit from their data collection processes need to learn, embrace, and implement digitalization in all its stages. (Part 2 of 3--Data Collection)
"Data is like garbage.You had better know what you are going to do with it before you collect it."--Attributed to author Mark Twain
The Internet of Things (IoT) is a major change in sensor data collection. This data needs to be stored efficiently so that it can be retrieved on demand for real-time applications. Most of the Cloud-IoT solutions focus on centralized data collection and storage which is not appropriate for efficient data collection and utilization. For addressing a such diverse set of requirements, resources are placed near to the data sources for processing and fast real-time decision making ["on the Edge"] instead of sending all the data to the Cloud.
Data collection on edge devices has the power to transform critical infrastructures such as oil, gas, energy, environment, and water. It is a pioneering tool in the transformation of smart city design. Technology adoption is key to enhancing the delivery and performance of infrastructure systems. It is no longer sufficient to collect data; future infrastructure solutions need to contain "smart" solutions.
Artificial intelligence (AI) and machine learning (ML) are integral parts of digital solutions that improve data quality by cleaning and improving the data and getting better-looking data sets through AI tools. To enhance real-time awareness and action, meaning that digital tools catch events in real-time leading to productive domain expertise, several challenges need to be addressed:
-
Power - Taking AI to edge devices at scale is blocked by power constraints. Edge devices are battery-powered, but assets have limited power sources in the field. Battery-powered edge devices must be capable of detecting data even in low-power modes. Servers need to be contacted at periodic intervals, and edge devices must have sufficient power reserves.
-
Connection - Connectivity to the external cloud is not welcome and, in some cases, it is not even allowed. This constraint necessitates an independent solution, i.e. data collection to edge devices. Instead of bringing field data to AI, it is now possible to apply AI in the field on edge devices.
-
Limited computational resources - There are no pre-processing or decision-making capabilities on edge devices that collect data from smart sensors. AI training of data sets is limited.
-
Security - Data collection must also be a cyber-secure process. Critical infrastructure is frequently hacked because bad actors know that service providers are willing to pay ransom.
Once these challenges have been addressed, the benefits of edge AI computing are accrued quickly. Edge-embedded AI is not like cloud-based AI. Edge devices enable immediate adoption of AI tools. They provide the benefits of AI without cloud connectivity. Real-time awareness and action become a reality as events are detected in real-time. This stands in contrast to traditional IoT that does not provide any additional value over the raw data; does not improve data quality and integrity; and real-time detection is not available.
Artificial intelligence is one of today's most disruptive trends in critical infrastructure. Most companies are shifting toward AI applications and attempting to figure out where they best meet the adoption of its capabilities. A more aggressive approach to adoption is predicted in the next few years. According to McKinsey, AI could deliver an additional economic activity of $13 trillion by 2030. In Part 3, data utilization will be discussed.
For Part 1 of this series on data, look here to learn about data creation: https://www.ayyeka.com/blog/without-data-just-opinion